Willard Ford, a senior at the University of California, San Diego (UCSD), is a rising star in the world of bioinformatics and data science. Majoring in Bioinformatics and Probability & Statistics, Willard’s research interests focus on genomic algorithms, statistical modeling, and population genetics. He is passionate about addressing healthcare inequities and improving patient outcomes, particularly through the application of personalized medicine powered by deep learning advancements.
Academic Background And Research Interests Of Willard Ford
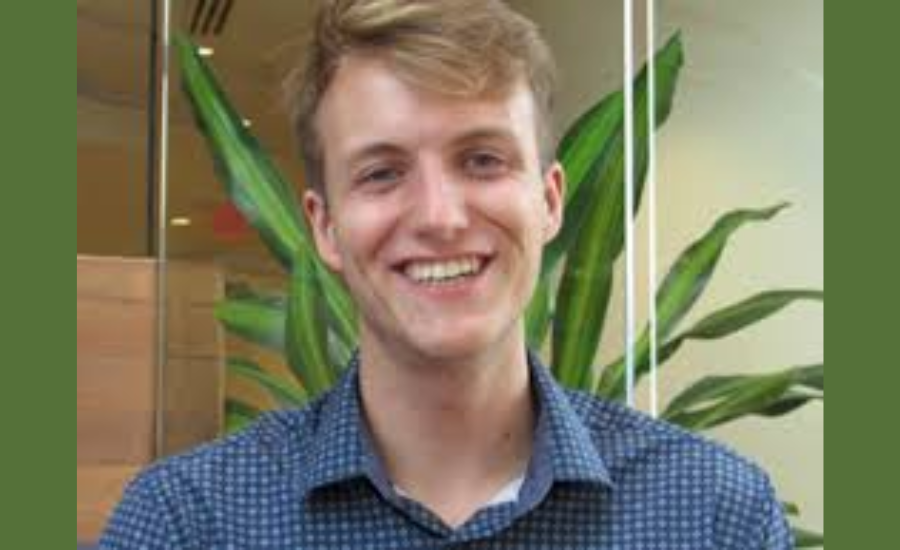
Double Major in Bioinformatics and Statistics
Willard is pursuing a double major in Bioinformatics and Probability & Statistics, a combination that gives him the tools to explore complex biological data with a statistical lens. These fields complement each other well in the world of genomic research, where data science and biological sciences intersect.
He is passionate about using statistical algorithms to solve problems in human genetics. His focus is on heritable diseases with unclear genetic causes. His commitment to healthcare equity drives him to find ways to make healthcare more accessible. He aims to use algorithms to improve care for underserved populations.
Focus on Personalized Medicine and Deep Learning
Willard’s enthusiasm for personalized medicine is fueled by the breakthroughs in deep learning and how it can be applied to healthcare. By utilizing patient-specific data, he believes that deep learning can lead to more accurate and efficient diagnoses and treatments. His goal is to develop algorithms that can help pinpoint the underlying causes of diseases that have been historically challenging to treat or understand, thus improving overall patient outcomes.
Groundbreaking Research On Structural Variants
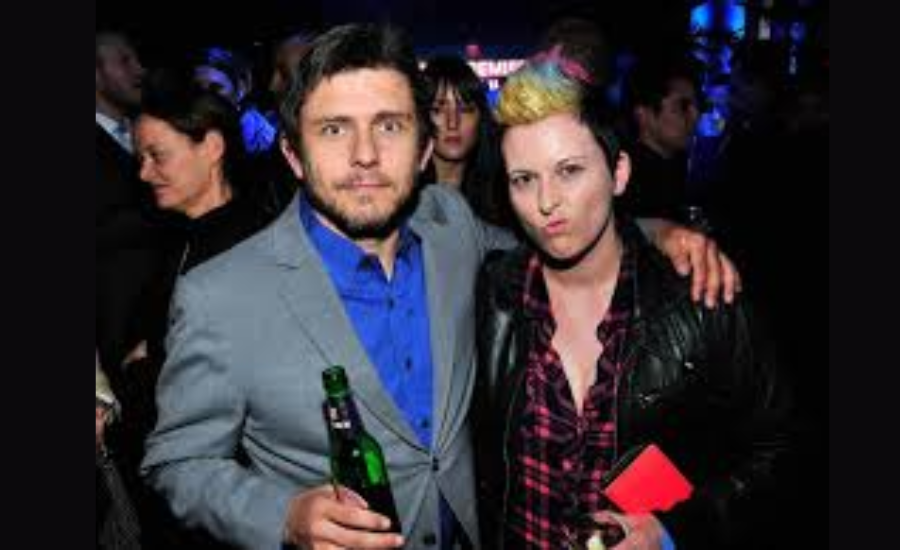
Understanding Structural Variants (SVs)
One of Willard’s major areas of research focuses on structural variants (SVs), large genomic alterations that involve the rearrangement of at least 50 consecutive bases in the DNA sequence. SVs are crucial for understanding heritable diseases, yet they remain difficult to detect using traditional sequencing methods.
Willard’s research in this field aims to unravel how these SVs contribute to complex diseases and genetic disorders. By leveraging cutting-edge sequencing technologies, he hopes to uncover the hidden genetic factors that contribute to the heritability gap in genetic diseases.
Research Experience in the BSRP Program
Willard’s time in the Biology Scholars Research Program (BSRP) was transformative in his scientific career. During this time, he explored genetic algorithms. He built tools and formed hypotheses with help from his mentors. Willard transitioned from a student to a scientist. He learned to ask meaningful scientific questions and test his own ideas.
Long-Read Sequencing and Its Challenges
One of the technologies at the forefront of Willard’s work is long-read sequencing, a method that allows researchers to sequence larger portions of the genome. While this technology offers the potential to explore hard-to-sequence regions, it comes at a high cost and presents significant computational challenges.
Willard’s research addresses these issues by optimizing SV detection tools, making it more feasible to work with large genomic cohorts without incurring excessive computational expenses.
Innovative Tools And Solutions
Optimizing SV Detection at Scale
Willard’s main project is Fast Structural Variant Merging at a Population Scale. It focuses on merging SVs from large datasets. His tools improve how SVs are identified and categorized. This leads to faster and more accurate analysis. It helps analyze genetic variations in thousands of samples.
Speeding Up Analysis with Efficient Filtering
One of the critical challenges in analyzing structural variants is the noise and redundancy in sequencing data. This excess information can obscure true disease associations, making it difficult to detect genetic markers for certain diseases.
Willard developed a method that uses hashing and sketching techniques to filter sequencing data, reducing the number of alignments needed by 62%. This innovation speeds up the analysis process without sacrificing accuracy. His solution preserves 95% of the original accuracy, allowing researchers to process large cohorts more efficiently and accurately.
Comparative Analysis of Filtering Strategies
Willard tested several filtering strategies, including length filtering, min-hashing, sketching, and Euclidean distance approximations. Additionally, his findings show that combining these methods yields better results. In contrast, using any single filtering technique on its own is less effective. Thus, combining these strategies enhances the accuracy of the analysis. This work paves the way for more robust, large-scale studies that could uncover novel genetic markers associated with structural variants and disease susceptibility.
Mentorship And Collaboration
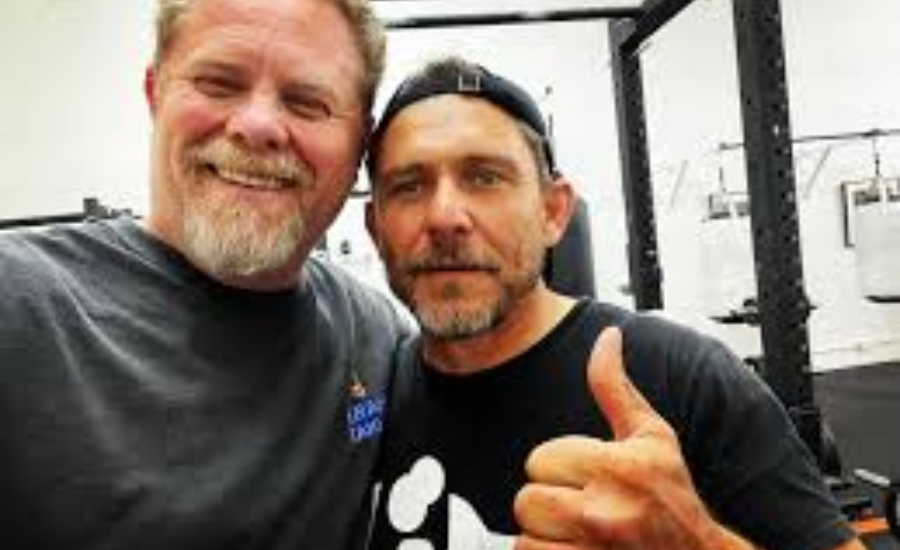
Support from Fabio Cunial and Ryan Lorig-Roach
Willard’s academic journey has been shaped by mentors Fabio Cunial and Ryan Lorig-Roach. They are experts in data science and population genetics. Their guidance has been crucial in Willard’s development. They provided support and tools for his SV research. Their mentorship helped Willard advance in understanding genetic variation. This mentorship has empowered him to tackle complex problems and develop practical solutions to real-world challenges in genomics.
Future Aspirations And Contributions Of Willard Ford
Advancing Bioinformatics and Healthcare
Looking ahead, Willard is determined to continue exploring the intersection of bioinformatics and healthcare. He aims to develop tools and algorithms that address the fundamental questions in genetics and disease association. With his passion for healthcare equity, he is driven to create more personalized, accessible medical solutions that cater to the needs of diverse populations.
Willard’s long-term goal is to contribute to the future of genomic medicine, where deep learning and data-driven insights can lead to improved healthcare outcomes for all individuals. His vision includes reducing healthcare disparities through targeted, data-driven approaches that focus on individual genetic profiles and healthcare needs.
Conclusion: A Bright Future Ahead
Willard Ford is an exceptionally talented individual with a strong passion for bioinformatics, genomics, and healthcare equity. His innovative research in structural variant detection and his exploration of new ways to process and analyze genomic data place him at the forefront of the field. As he continues his academic journey, Willard is gaining valuable experience and developing his research skills. Consequently, he is poised to make a lasting impact on genetic research and personalized medicine. With his determination and mentorship, Willard is steadily advancing toward his goals. Moreover, his vision for the future suggests that his contributions will significantly shape healthcare and genomics. Therefore, Willard’s future work could transform the field in the coming years.
FAQS About Willard Ford
1. Who is Willard Ford?
Willard Ford is a senior at the University of California, San Diego (UCSD), where he is pursuing a double major in Bioinformatics and Probability & Statistics. He is passionate about using genomic algorithms and statistical modeling to address challenges in population genetics and healthcare inequities. His research focuses on understanding structural variants (SVs) in the genome and exploring personalized medicine through deep learning advancements.
2. What is Willard Ford’s main area of research?
Willard’s main research focus is on structural variants (SVs), which are large genomic alterations that affect at least 50 consecutive bases of DNA. These SVs are associated with various diseases, but they are difficult to detect using traditional genetic sequencing methods. As a result, Willard is developing new tools to detect and analyze these SVs more efficiently. Specifically, he is concentrating on large genomic cohorts to tackle this challenge. To achieve this, Willard employs advanced techniques like hashing and sketching, which enhance the detection process. Consequently, his work is significantly improving the detection and analysis of these complex genetic variations.
3. What is the significance of Willard Ford’s research?
Willard’s research aims to improve the detection of structural variants, which could help identify the genetic causes of diseases that have not been fully explained by traditional sequencing methods. By optimizing the computational efficiency of SV detection, his work enables large-scale studies that could uncover novel disease associations and lead to better understanding and treatment of genetic disorders.
4. How does Willard Ford’s work impact healthcare?
Willard’s work has the potential to revolutionize healthcare by improving the accuracy and efficiency of genomic medicine. Through deep learning and personalized medicine, his research aims to provide tailored treatments for individuals based on their unique genetic profiles. Additionally, by focusing on healthcare equity, Willard strives to address the disparities in healthcare access and outcomes for underserved populations.
5. What are structural variants (SVs) and why are they important?
Structural variants (SVs) are large genetic alterations, such as deletions, duplications, or rearrangements of segments of DNA. These variants can impact up to 50 or more consecutive bases of the genome and are associated with various diseases. SVs are difficult to detect with traditional sequencing techniques, but they are essential to understanding genetic diseases that are otherwise unexplained.
Discover Engaging Insights and Trends at vents.co.